Generalized data-driven model-free predictive control designed for electrical drive systems
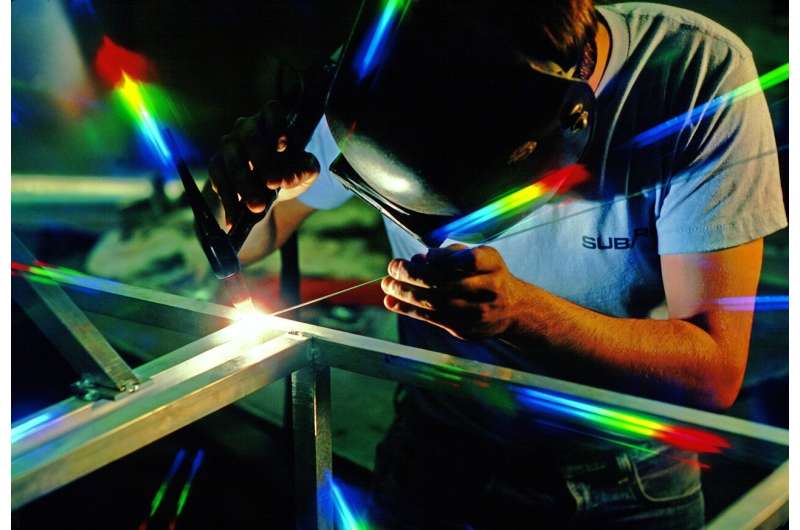
High-end equipment manufacturing has experienced rapid development in recent years, and the alternating current (AC) motor is indispensable. Traditional control strategies on the motor driving system cannot fully meet the requirements such as strict control accuracy, stable torque and multiple objective optimizations. As the third generation of advanced control technology, predictive control has attracted great attention for its excellent dynamic performance and control accuracy.
Since the physical parameters of the motor are nonlinearly changing, the motor driving system continuously operates under the condition of parameter mismatch. However, the predictive control has weak robustness because of the heavy dependence on the model accuracy for the achieved performance, so its application in the high-end manufacturing field is restricted. A data-driven model is adopted in the model-free predictive control strategy to essentially eliminate the influences of the parameter mismatches and enhance the robustness.
In a study published in IEEE Transactions on Industrial Electronics, Prof. Wang Fengxiang’s group from Fujian Institute of Research on the Structure of Matter of the Chinese Academy of Sciences designed an auto-regressive with exogenous input (ARX) model based on the recursive least square (RLS) estimation algorithm to accurately fit the motor online.
The researchers analyzed the structure of the data-driven model. Considering the operating states during the past sampling periods, they designed an ARX data-driven model to satisfy the characteristics of the mechanical part in the motor. ARX is a fractional expression and its denominator and numerator are discrete-time transfer functions by a group of time series of the sampled currents, in which the coefficients in the transfer functions are online selected to fit the plant accurately.
Then, they estimated the above-mentioned coefficients based on the RLS algorithm, in which the regressor vectors gathering the sampled data and coefficients are updated at each sampling period, and the predictive variables are obtained by time-shift. Intriguingly, the convergence rate of the coefficients is slower compared with that of the system, and this process is little influenced by the disturbances.
The researchers found that the orders of the transfer function are also important values determining the control performance directly. More complex operating states of the system can be accurately fitted by the data-driven model with increased orders. However, these values are always restricted by processor resources, and this term becomes a potential optimization.
The researchers selected a permanent magnet synchronous motor (PMSM) speed control system as an example to demonstrate the effectiveness of the presented control strategy. According to the simulation and experimental results, the presented control strategy obtains improved dynamics, current quality and robustness compared to the conventional model-free predictive control based on ultra-local under the same conditions.
Besides, the presented method has enough compatibility to be applied to other motor driving systems due to the generalized structure of the data-driven model.
This study provides an essential guidance for the future design and synthesis of model-free predictive control for the motor driving system.
Robust predictive speed control for surface-mounted permanent magnet synchronous motor systems
Yao Wei et al, Generalized Data-Driven Model-Free Predictive Control for Electrical Drive Systems, IEEE Transactions on Industrial Electronics (2022). DOI: 10.1109/TIE.2022.3210563
Citation:
Generalized data-driven model-free predictive control designed for electrical drive systems (2022, October 18)
retrieved 18 October 2022
from https://techxplore.com/news/2022-10-data-driven-model-free-electrical.html
This document is subject to copyright. Apart from any fair dealing for the purpose of private study or research, no
part may be reproduced without the written permission. The content is provided for information purposes only.
For all the latest Technology News Click Here
For the latest news and updates, follow us on Google News.