Detecting additive manufacturing defects in real-time
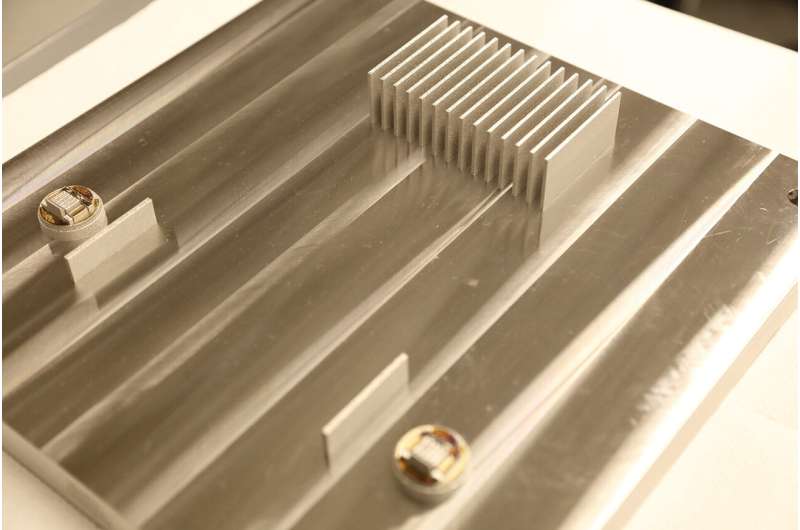
A research team led by Tao Sun, associate professor of materials science and engineering at the University of Virginia, has made new discoveries that can expand additive manufacturing in aerospace and other industries that rely on strong metal parts.
Their peer-reviewed paper was published Jan. 6, 2023, in Science. It addresses the issue of detecting the formation of keyhole pores, one of the major defects in a common additive manufacturing technique called laser powder bed fusion, or LPBF.
Introduced in the 1990s, LPBF uses metal powder and lasers to 3-D print metal parts. But porosity defects remain a challenge for fatigue-sensitive applications like aircraft wings. Some porosity is associated with deep and narrow vapor depressions which are the keyholes.
The formation and size of the keyhole is a function of laser power and scanning velocity, as well as the materials’ capacity to absorb laser energy. If the keyhole walls are stable, it enhances the surrounding material’s laser absorption and improves laser manufacturing efficiency. If, however, the walls are wobbly or collapse, the material solidifies around the keyhole, trapping the air pocket inside the newly formed layer of material. This makes the material more brittle and more likely to crack under environmental stress.
Sun and his team, including materials science and engineering professor Anthony Rollett from Carnegie Mellon University and mechanical engineering professor Lianyi Chen from the University of Wisconsin-Madison, developed an approach to detect the exact moment when a keyhole pore forms during the printing process.
“By integrating operando synchrotron X-ray imaging, near-infrared imaging, and machine learning, our approach can capture the unique thermal signature associated with keyhole pore generation with sub-millisecond temporal resolution and 100% prediction rate,” Sun said.
In developing their real-time keyhole detection method, the researchers also advanced the way a state-of-the-art tool—operando synchrotron X-ray imaging—can be used. Utilizing machine learning, they additionally discovered two modes of keyhole oscillation.
“Our findings not only advance additive manufacturing research, but they can also practically serve to expand the commercial use of LPBF for metal parts manufacturing,” said Rollett. Rollet is also the co-director of the NextManufacturing Center at CMU.
“Porosity in metal parts remains a major hurdle for wider adoption of LPBF technique in some industries. Keyhole porosity is the most challenging defect type when it comes to real-time detection using lab-scale sensors because it occurs stochastically beneath the surface,” Sun said. “Our approach provides a viable solution for high-fidelity, high-resolution detection of keyhole pore generation that can be readily applied in many additive manufacturing scenarios.”
Zhongshu Ren et al, Machine learning–aided real-time detection of keyhole pore generation in laser powder bed fusion, Science (2023). DOI: 10.1126/science.add4667
Citation:
Detecting additive manufacturing defects in real-time (2023, January 6)
retrieved 6 January 2023
from https://techxplore.com/news/2023-01-additive-defects-real-time.html
This document is subject to copyright. Apart from any fair dealing for the purpose of private study or research, no
part may be reproduced without the written permission. The content is provided for information purposes only.
For all the latest Technology News Click Here
For the latest news and updates, follow us on Google News.