Data collected from acquaintances and even strangers can predict your location
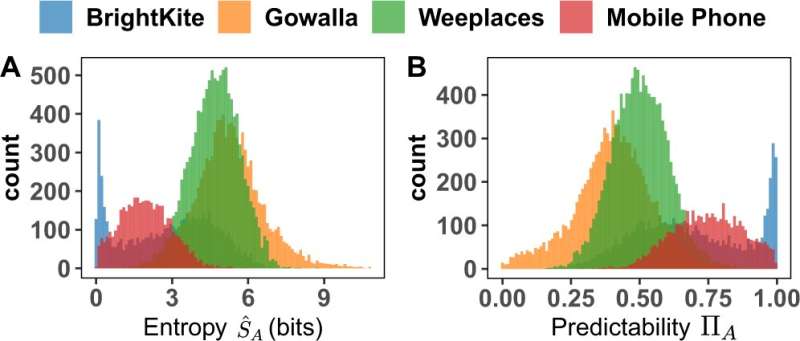
Data about our habits and movements are constantly collected via mobile phone apps, fitness trackers, credit card logs, websites visited, and other means.
But if we turn off data tracking on our devices, aren’t we untraceable?
No, according to a new study.
“Switching off your location data is not going to entirely help,” says Gourab Ghoshal, an associate professor of physics, mathematics, and computer science and the Stephen Biggar ’92 and Elizabeth Asaro ’92 Fellow in Data Science at the University of Rochester.
Ghoshal, joined by colleagues at the University of Exeter, the Federal University of Rio de Janeiro, Northeastern University, and the University of Vermont, applied techniques from information theory and network science to find out just how far-reaching a person’s data might be. The researchers discovered that even if individual users turned off data tracking and didn’t share their own information, their mobility patterns could still be predicted with surprising accuracy based on data collected from their acquaintances.
“Worse,” says Ghoshal, “almost as much latent information can be extracted from perfect strangers that the individual tends to co-locate with.”
The researchers published their findings in Nature Communications.
The smoking gun: Your ‘colocation network’
The researchers analyzed four datasets: three location-based social network datasets composed of millions of check-ins on apps such as Brightkite, Facebook, and Foursquare, and one call-data record containing more than 22 million calls by nearly 36,000 anonymous users.
They developed a “colocation” network to distinguish between the mobility patterns of two sets of people:
- people who are socially tied to an individual, such as family members, friends, or co-workers
- people who are not socially tied to an individual, but who are at a location at a similar time as the individual. They might include people working in the same building but with different companies, parents whose children attend the same schools but who are unknown to each other, or people who shop at the same grocery store.
By applying information theory and measures of entropy—the degree of randomness or structure in a sequence of location visits—the researchers learned that the movement patterns of people who are socially tied to an individual contain up to 95% of the information needed to predict that individual’s mobility patterns. However, even more surprisingly, they found that strangers not tied socially to an individual could also provide significant information, predicting up to 85% of an individual’s movement.
‘A cautionary tale’
The ability to predict the locations of individuals or groups can be beneficial in areas such as urban planning and pandemic control, where contact tracing based on mobility patterns is a key tool to stopping the spread of disease. In addition, many consumers appreciate the ability of data mining to offer tailored recommendations for restaurants, TV shows, and advertisements.
However, Ghoshal says, data mining is a slippery slope, especially because, as the research showed, individuals sharing data via mobile apps may be unwittingly providing information about others.
“We’re offering a cautionary tale that people should be aware of how far-reaching their data can be,” he says. “This research has a lot of implications for surveillance and privacy issues, especially with the rise of authoritarian impulses. We can’t just tell people to switch off their phones or go off the grid. We need to have dialogs to put in place laws and guidelines that regulate how people collecting your data use it.”
Zexun Chen et al, Contrasting social and non-social sources of predictability in human mobility, Nature Communications (2022). DOI: 10.1038/s41467-022-29592-y
Citation:
Data collected from acquaintances and even strangers can predict your location (2022, April 13)
retrieved 13 April 2022
from https://techxplore.com/news/2022-04-acquaintances-strangers.html
This document is subject to copyright. Apart from any fair dealing for the purpose of private study or research, no
part may be reproduced without the written permission. The content is provided for information purposes only.
For all the latest Technology News Click Here
For the latest news and updates, follow us on Google News.