An architecture that combines deep neural networks and vector-symbolic models
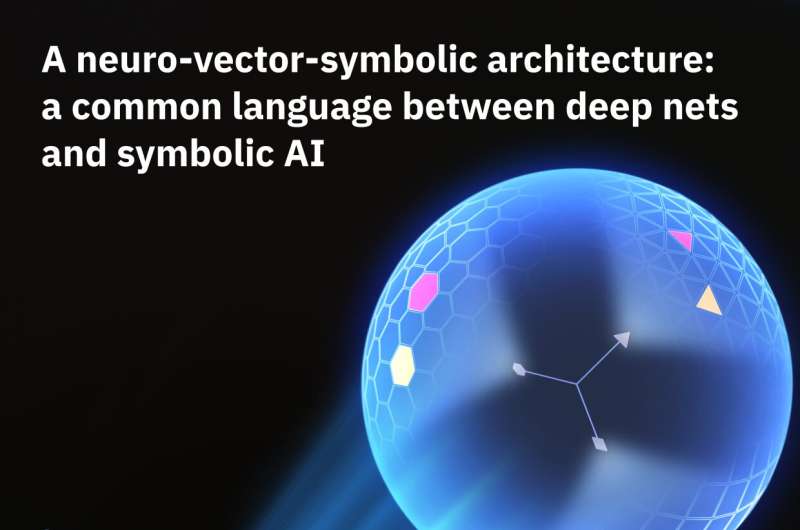
Researchers at IBM Research Zürich and ETH Zürich have recently created a new architecture that combines two of the most renowned artificial intelligence approaches, namely deep neural networks and vector-symbolic models. Their architecture, presented in Nature Machine Intelligence, could overcome the limitations of both these approaches, solving progressive matrices and other reasoning tasks more effectively.
“Our recent paper was based on our earlier research works aimed at augmenting and enhancing neural networks with the powerful machinery of vector-symbolic architectures (VSAs),” Abbas Rahimi, one of the researchers who carried out a study, told Tech Xplore. “This combination was previously applied to few-shot learning as well as few-shot continual learning tasks, achieving state-of-the-art accuracy with lower computational complexity. In our recent paper, we take this concept beyond perception, by focusing on solving visual abstract reasoning tasks, specifically, the widely used IQ tests known as Raven’s progressive matrices.”
Raven’s progressive matrices are non-verbal tests typically used to test people’s IQ and abstract reasoning skills. They consist in a series of items presented in sets, where one or more item is missing.
To solve Raven’s progressive matrices, respondents need to correctly identify the missing items in given sets among a few possible choices. This requires advanced reasoning capabilities, such as being able to detect abstract relationships between objects, which could be related to their shape, size, color, or other features.
The neuro-vector-symbolic architecture (NVSA) developed by Rahimi and his colleagues combines deep neural networks, which are known to perform well on perception tasks, with VSA machinery. VSAs are unique computational models that perform symbolic computations using distributed, high-dimensional vectors.
“While our approach might sound a bit like neuro-symbolic AI approaches, neuro-symbolic AI has inherited the limitations of their individual deep learning and classical symbolic AI components,” Rahimi explained. “Our key objective is to address these limitations, namely the neural binding problem and exhaustive search, in NVSA by using a common language between the neural and symbolic components.”
The team’s combination of deep neural networks and VSAs was supported by two main architecture design features. These include a new neural network training process and a method to perform VSA transformations.
“We developed two key enablers of our architecture,” Rahimi said. “The first is the use of a novel neural network training method as a flexible means of representation learning over VSA. The second is a method to attain proper VSA transformations such that exhaustive probability computations and searches can be substituted by simpler algebraic operations in the VSA vector space.”
In initial evaluations, the architecture developed by Rahimi and his colleagues attained very promising results, solving Raven’s progressive matrices faster and more efficiently than other architectures developed in the past. Specifically, it performed better than both state-of-the-art deep neural networks and neuro-symbolic AI approaches, achieving new record accuracies of 87.7% on the RAVEN dataset and 88.1% on the I-RAVEN dataset.
“To solve a Raven test, something called probabilistic abduction is required, a process that involves searching for a solution in a space defined by prior background knowledge about the test,” Rahimi said. “The prior knowledge is represented in symbolic form by describing all possible rule realizations that could govern the Raven tests. The purely symbolic reasoning approach needs to go through all valid combinations, compute the rule probability, and sum them up. This search becomes a computational bottleneck in the large search space, due to a large number of combinations that would be prohibitive to test.”
In contrast with existing architectures, NVSA can perform extensive probabilistic calculations in a single vector operation. This in turn allows it to solve abstract reasoning and analogy-related problems, such as Raven’s progressive matrices, faster and more accurately than other AI approaches based on deep neural networks or VSAs alone.
“Our approach also addresses the neural binding problem, enabling a single neural network to separately recognize distinct properties of multiple objects simultaneously in a scene,” Rahimi said. “Overall, NVSA offers transparent, fast and efficient reasoning; and it is the very first example showing how probabilistic reasoning (as an upgrade of pure logical reasoning) can be efficiently performed by distributed representations and operators of VSA. Compared to the symbolic reasoning of neuro-symbolic approaches, the probabilistic reasoning of NVSA is two orders of magnitude faster, with less expensive operations on the distributed representations.”
The new architecture created by this team has so far proved to be highly promising for efficiently and rapidly solving complex reasoning tasks. In the future, it could be tested and applied to various other problems, while also potentially inspiring the development of other similar approaches.
“NVSA is an important step towards encapsulating different AI paradigms in a unified framework to address tasks involving both perception and higher-level reasoning,” Rahimi added. “Interestingly, NVSA already exhibited degrees of generalization to various unseen combinations of objects and object attributes. Further generalization in this direction is still an open problem.”
More information:
Michael Hersche et al, A neuro-vector-symbolic architecture for solving Raven’s progressive matrices, Nature Machine Intelligence (2023). DOI: 10.1038/s42256-023-00630-8
© 2023 Science X Network
Citation:
An architecture that combines deep neural networks and vector-symbolic models (2023, March 30)
retrieved 30 March 2023
from https://techxplore.com/news/2023-03-architecture-combines-deep-neural-networks.html
This document is subject to copyright. Apart from any fair dealing for the purpose of private study or research, no
part may be reproduced without the written permission. The content is provided for information purposes only.
For all the latest Technology News Click Here
For the latest news and updates, follow us on Google News.