Team-knowledge distillation for multiple cross-domain, few-shot learning
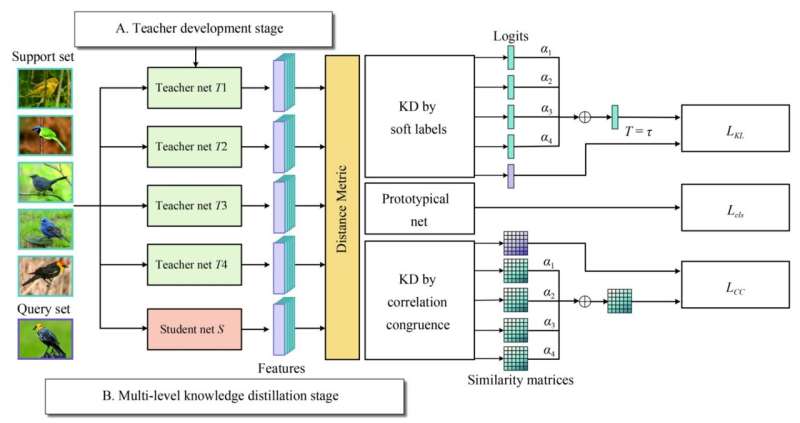
Although few-shot learning (FSL) has achieved great progress, it is still an enormous challenge especially when the source and target sets are from different domains, which is also known as cross-domain few-shot learning (CD-FSL). Utilizing more source domain data is an effective way to improve the performance of CD-FSL. However, knowledge from different source domains may entangle and confuse with each other, which hurts the performance on the target domain.
A research team led by professor Zhong JI in Tianjin University published their new research on March 27, 2023 in Frontiers of Computer Science.
The team propose team-knowledge distillation networks (TKD-Net) to tackle the CD-FSL, which explores a strategy to help the cooperation of multiple teachers. They distill knowledge from the cooperation of teacher networks to a single student network in a meta-learning framework. It incorporates task-oriented knowledge distillation and multiple cooperation among teachers to train an efficient student with better generalization ability on unseen tasks. Moreover, their TKD-Net employs both response-based knowledge and relation-based knowledge to transfer more comprehensive and effective knowledge.
Specifically, their proposed method consists of two stages: a teacher development stage and a multi-level knowledge distillation stage. They first respectively pre-train teacher models with the training data from multiple seen domains by supervised learning, where all the teacher models have the same network architecture. After obtaining multiple domain-specific teacher models, multi-level knowledge is then transferred from the cooperation of teachers to the student in the paradigm of meta-learning.
Task-oriented distillation is beneficial for the student model to quickly adapt to few-shot tasks. The student model is trained based on Prototypical Networks and the soft labels provided by the teacher models. Additionally, they further explore the knowledge embedded in the similarity and explore the similarity matrix of teachers to transfer the relationship between samples in the few-shot tasks. It guides the student to learn more specific and comprehensive information.
Future work may focus on adaptively adjusting the weight of multiple teacher models, and finding more ways to effectively aggregate the knowledge from multiple teachers.
More information:
Zhong Ji et al, Teachers cooperation: team-knowledge distillation for multiple cross-domain few-shot learning, Frontiers of Computer Science (2022). DOI: 10.1007/s11704-022-1250-2
Provided by
Frontiers Journals
Citation:
Team-knowledge distillation for multiple cross-domain, few-shot learning (2023, May 15)
retrieved 15 May 2023
from https://techxplore.com/news/2023-05-team-knowledge-distillation-multiple-cross-domain-few-shot.html
This document is subject to copyright. Apart from any fair dealing for the purpose of private study or research, no
part may be reproduced without the written permission. The content is provided for information purposes only.
For all the latest Technology News Click Here
For the latest news and updates, follow us on Google News.