Neural network analyzes gravitational waves in real time
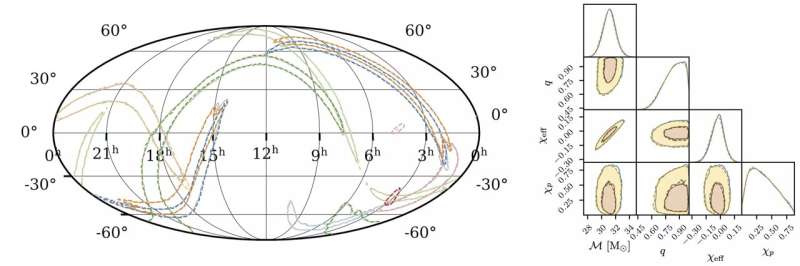
Black holes are one of the greatest mysteries of the universe—for example, a black hole with the mass of our sun has a radius of only 3 kilometers. Black holes in orbit around each other emit gravitational radiation—oscillations of space and time predicted by Albert Einstein in 1916. This causes the orbit to become faster and tighter, and eventually, the black holes merge in a final burst of radiation. These gravitational waves propagate through the universe at the speed of light, and are detected by observatories in the U.S. (LIGO) and Italy (Virgo). Scientists compare the data collected by the observatories against theoretical predictions to estimate the properties of the source, including how large the black holes are and how fast they are spinning. Currently, this procedure takes at least hours, often months.
An interdisciplinary team of researchers from the Max Planck Institute for Intelligent Systems (MPI-IS) in Tübingen and the Max Planck Institute for Gravitational Physics (Albert Einstein Institute/AEI) in Potsdam is using state-of-the-art machine learning methods to speed up this process. They developed an algorithm using a deep neural network, a complex computer code built from a sequence of simpler operations, inspired by the human brain. Within seconds, the system infers all properties of the binary black-hole source. Their research results are published today in Physical Review Letters.
“Our method can make very accurate statements in a few seconds about how big and massive the two black holes were that generated the gravitational waves when they merged. How fast do the black holes rotate, how far away are they from Earth and from which direction is the gravitational wave coming? We can deduce all this from the observed data and even make statements about the accuracy of this calculation,” explains Maximilian Dax, first author of the study Real-Time Gravitational Wave Science with Neural Posterior Estimation and Ph.D. student in the Empirical Inference Department at MPI-IS.
The researchers trained the neural network with many simulations—predicted gravitational-wave signals for hypothetical binary black-hole systems combined with noise from the detectors. This way, the network learns the correlations between the measured gravitational-wave data and the parameters characterizing the underlying black-hole system. It takes ten days for the algorithm called DINGO (the abbreviation stands for Deep INference for Gravitational-wave Observations) to learn. Then it is ready for use: the network deduces the size, the spins, and all other parameters describing the black holes from data of newly observed gravitational waves in just a few seconds. The high-precision analysis decodes ripples in space-time almost in real-time—something that has never been done with such speed and precision. The researchers are convinced that the improved performance of the neural network as well as its ability to better handle noise fluctuations in the detectors will make this method a very useful tool for future gravitational-wave observations.
“The further we look into space through increasingly sensitive detectors, the more gravitational-wave signals are detected. Fast methods such as ours are essential for analyzing all of this data in a reasonable amount of time,” says Stephen Green, senior scientist in the Astrophysical and Cosmological Relativity department at the AEI. “DINGO has the advantage that—once trained—it can analyze new events very quickly. Importantly, it also provides detailed uncertainty estimates on parameters, which have been hard to produce in the past using machine-learning methods.”
Until now, researchers in the LIGO and Virgo collaborations have used computationally very time-consuming algorithms to analyze the data. They need millions of new simulations of gravitational waveforms for the interpretation of each measurement, which leads to computing times of several hours to months—DINGO avoids this overhead because a trained network does not need any further simulations for analyzing newly observed data, a process known as “amortized inference.”
The method holds promise for more complex gravitational-wave signals describing binary—black-hole configurations, whose use in current algorithms makes analyses very time-consuming, and for binary neutron stars. Whereas the collision of black holes releases energy exclusively in the form of gravitational waves, merging neutron stars also emit radiation in the electromagnetic spectrum. They are therefore also visible to telescopes which have to be pointed to the respective region of the sky as quickly as possible in order to observe the event. To do this, one needs to very quickly determine where the gravitational wave is coming from, as facilitated by the new machine learning method. In the future, this information could be used to point telescopes in time to observe electromagnetic signals from the collisions of neutron stars, and of a neutron star with a black hole.
Alessandra Buonanno, director at the AEI, and Bernhard Schölkopf, director at the MPI-IS says, “Going forward, these approaches will also enable a much more realistic treatment of the detector noise and of the gravitational signals than is possible today using standard techniques.”
Schölkopf says, “Simulation-based inference using machine learning could be transformative in many areas of science where we need to infer a complex model from noisy observations.”
Maximilian Dax et al, Real-Time Gravitational Wave Science with Neural Posterior Estimation, Physical Review Letters (2021). DOI: 10.1103/PhysRevLett.127.241103
Citation:
Neural network analyzes gravitational waves in real time (2021, December 10)
retrieved 10 December 2021
from https://techxplore.com/news/2021-12-neural-network-gravitational-real.html
This document is subject to copyright. Apart from any fair dealing for the purpose of private study or research, no
part may be reproduced without the written permission. The content is provided for information purposes only.
For all the latest Technology News Click Here
For the latest news and updates, follow us on Google News.