LaundroGraph: Using deep learning to support anti-money laundering efforts
November 24, 2022
feature
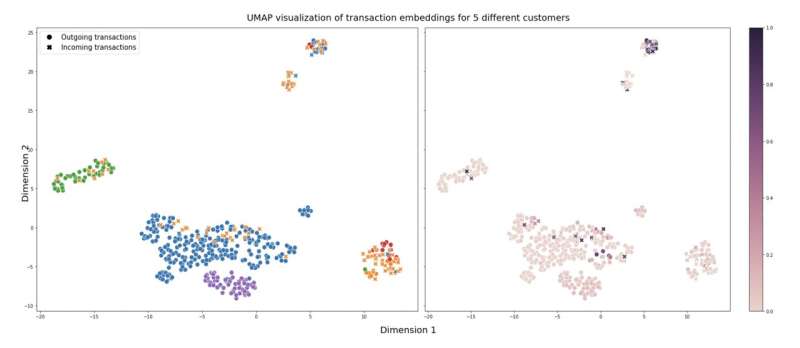
In recent years, deep learning techniques have proved to be highly valuable for tackling countless research and real-world problems. Researchers at Feedzai, a financial data science company based in Portugal, have demonstrated the potential of deep learning for the prevention and detection of illicit money laundering activities.
In a paper presented at the 3rd ACM International Conference on AI in Finance, the team at Feedzai introduced LaundroGraph, a self-supervised model that could simplify the cumbersome process of reviewing large amounts of financial interactions looking for suspicious transactions or monetary exchanges. Their model is based on a graph neural network, an artificial neural network (ANN) designed to autonomously process large amounts of data that can be represented as a graph.
“Wanting to strengthen our AML solution, and after identifying major pains with the current AML reviewing process, we thought about solutions to overcome these challenges using AI,” Mario Cardoso, a Research Data Scientist at Feedzai, told TechXplore.
“AML is particularly challenging due to label scarcity and the fact that the context surrounding the financial movements, specifically the entities interacted with and the properties of each transaction, are crucial to inform decisions. With these challenges in mind, we sought to create a machine learning approach that can support human analysts and facilitate AML reviewing.”
Reviewing financial interactions looking for suspicious activity can be a very tedious and time-consuming task for human analysts. Cardoso and his colleagues set out to greatly simplify this task using deep learning techniques, which are known to be particularly good at analyzing large amounts of data.
LaundroGraph, the model they created, can encode banking customers and financial transactions, transforming them into meaningful graph representations. These representations can guide the work of anti-money laundering analysts, highlighting anomalous money movements for specific customers without them having to look at entire transaction histories.
“LaundroGraph generates dense, context-aware representations of behavior decoupled from any specific labels,” Cardoso explained. “It does so by exploiting both the structural and feature information of a graph through a link prediction task between customers and transactions. We define our graph as a customer-transaction bipartite graph, which we create using the raw financial movements data.”
The researchers at Feedzai evaluated their model in a series of tests, assessing its ability to predict suspicious transfers in a dataset of real-world transactions. They found that its prediction power was significantly higher than that of other baseline methods designed to support anti-money laundering efforts.
“Given that it requires no labels, LaundroGraph is suitable for a wide variety of real-world financial applications that could benefit from graph-structured data,” Cardoso said. “Our paper proposes to leverage these embeddings to provide insights that can accelerate the reviewing process of AML detection, but this approach can be extended to other use-cases (e.g., fraud) and the embeddings can serve a wide variety of purposes beyond the insights we analyze (e.g., feature enrichers).”
In the future, LaundroGraph could assist financial analysts and anti-money laundering agents worldwide with reviewing large amounts of financial transactions, helping them to identify anomalous activities more rapidly and efficiently. Cardoso and his colleagues are now planning to develop their model further, while also exploring its potential for solving other financial problems.
“Future directions for our research will include experimentation in additional use-cases, such as fraud, and research into other insights/tasks that can be enabled or enhanced through the embeddings, for example, using the embeddings as an informative starting point for a label-scarce downstream predictions,” Cardoso added.
Mário Cardoso et al, LaundroGraph: Self-Supervised Graph Representation Learning for Anti-Money Laundering, 3rd ACM International Conference on AI in Finance (2022). DOI: 10.1145/3533271.3561727
© 2022 Science X Network
Citation:
LaundroGraph: Using deep learning to support anti-money laundering efforts (2022, November 24)
retrieved 24 November 2022
from https://techxplore.com/news/2022-11-laundrograph-deep-anti-money-laundering-efforts.html
This document is subject to copyright. Apart from any fair dealing for the purpose of private study or research, no
part may be reproduced without the written permission. The content is provided for information purposes only.
For all the latest Technology News Click Here
For the latest news and updates, follow us on Google News.