Deep learning methods help solve power issues in MIMO technology
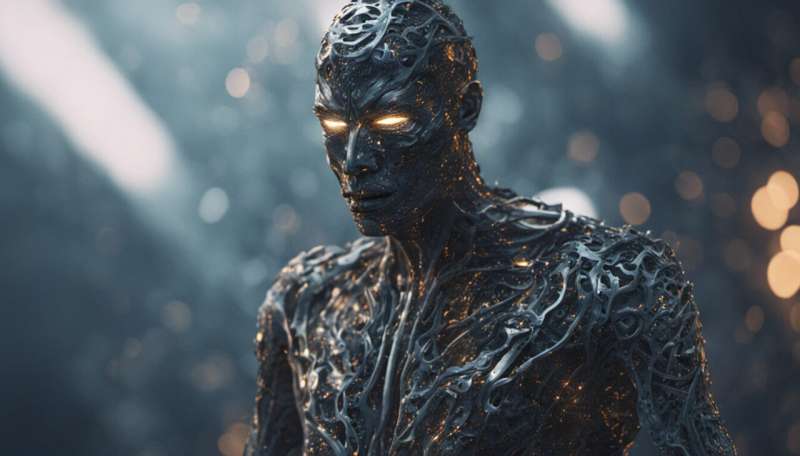
As of 2016, new 5G radio-access technology known as New Radio has been standardized by the 3rd Generation Partnership Project (3GPP) to satisfy the service demands of a wide range of applications such as Internet of Things (IoT), autonomous driving, and Virtual Reality (VR). The first set of 5G New Radio specifications has been released in the so-called 3GPP Release 15. Despite the advances already made, a number of issues remain, in particular regarding the demand for higher data rates, lower latencies, and improved robustness. For his Ph.D. research, Yu Zhao addressed power allocation challenges in 5G New Radio technologies by turning to deep learning methods.
Two key elements that play an essential role in addressing the challenges of 5G New Radio are the use of mmWave spectrum and massive multiple-input massive multiple-output (MIMO) technology. On the one hand, much more bandwidth is available in the mmWave spectrum than in the sub-6 GHz spectrum which results in higher data rates and, on the other hand, massive MIMO enables a high degree of space division multiplexing, which increases the network capacity for a given spectrum.
Recently, cell-free (CF) massive MIMO, has been proposed to further improve the spectral efficiency (SE) and hence the network capacity of the system. In CF massive MIMO, a number of access points (APs) with multiple antennas are spread over the coverage area and are connected to a central controller (CC) via a fronthaul. The APs jointly and coherently provide service to the user equipments (UEs). Compared to massive MIMO, CF massive MIMO is more robust against shadow fading and has a lower average distance between the transmitters and the receivers.
Geographical spreading
Due to the geographical spreading of antennas, local signal processing is performed at each AP, e.g., by using conjugate beamforming (CB), thereby avoiding the exchange of channel state information (CSI) between the CC and the APs. However, by doing this, the interference between different UEs cannot be suppressed, as would be the case when using zero-forcing beamforming (ZFB). Under these circumstances, the optimization of power allocation becomes non-convex and hence computationally hard.
For his thesis, Yu Zhao addressed three challenging power allocation problems: Problem 1, max-min power allocation in CF sub-6 GHz massive MIMO; Problem 2, max-sum SE power allocation in CF sub-6 GHz massive MIMO; Problem 3, max-sum SE power allocation in CF mmWave massive MIMO.
For Problem 1, he proposed the use of deep supervised learning (DSL). To address problem 2, deep reinforcement learning (DRL) was employed, and for Problem 3, he used both the deep Q-network method and deep deterministc policy gradient method.
The results of the Monte Carlo simulations show that the performance of the proposed methods is better. Moreover, in comparison to previous simulations, the execution time is drastically decreased.
Citation:
Deep learning methods help solve power issues in MIMO technology (2022, February 1)
retrieved 1 February 2022
from https://techxplore.com/news/2022-02-deep-methods-power-issues-mimo.html
This document is subject to copyright. Apart from any fair dealing for the purpose of private study or research, no
part may be reproduced without the written permission. The content is provided for information purposes only.
For all the latest Technology News Click Here
For the latest news and updates, follow us on Google News.