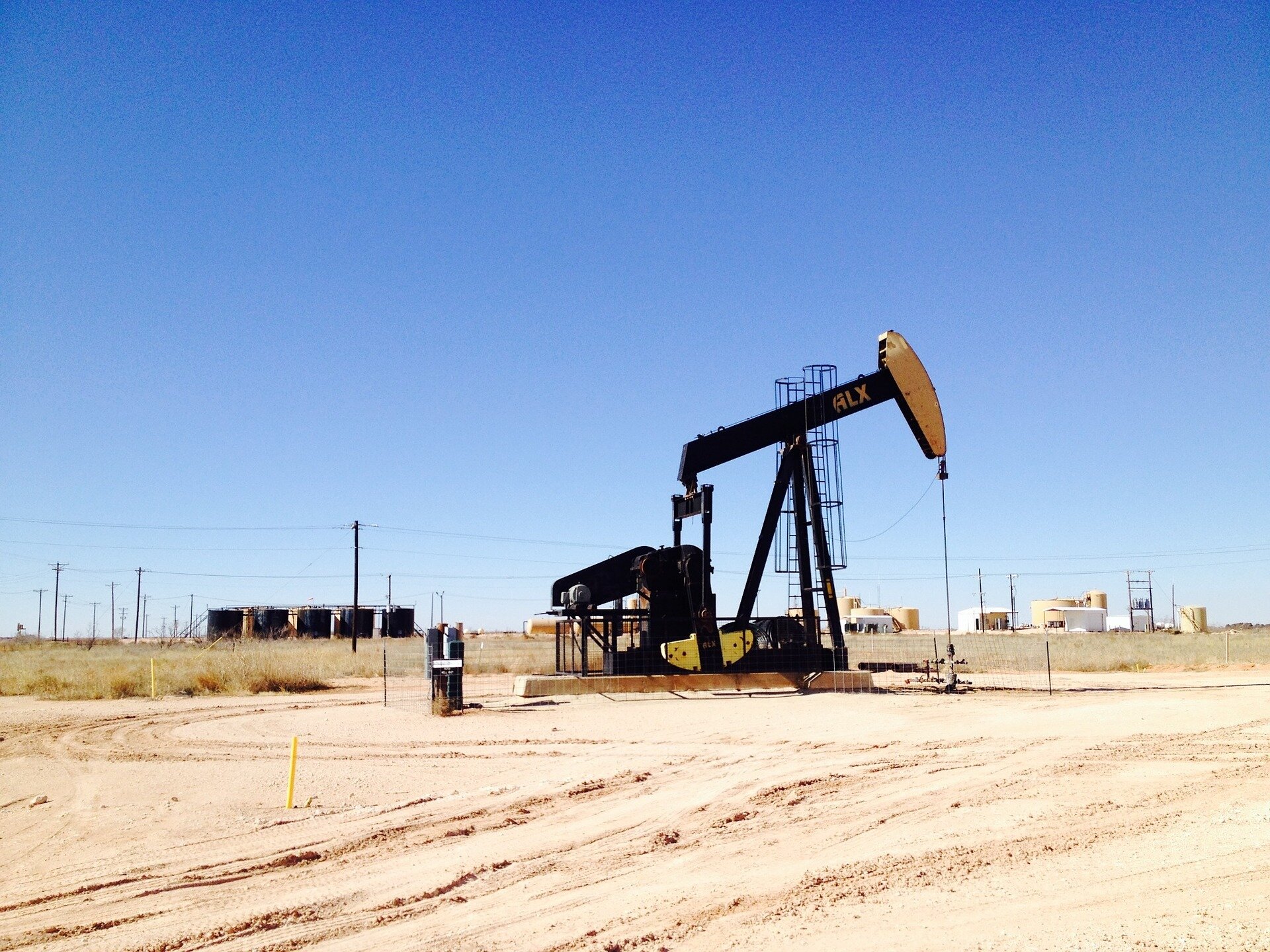
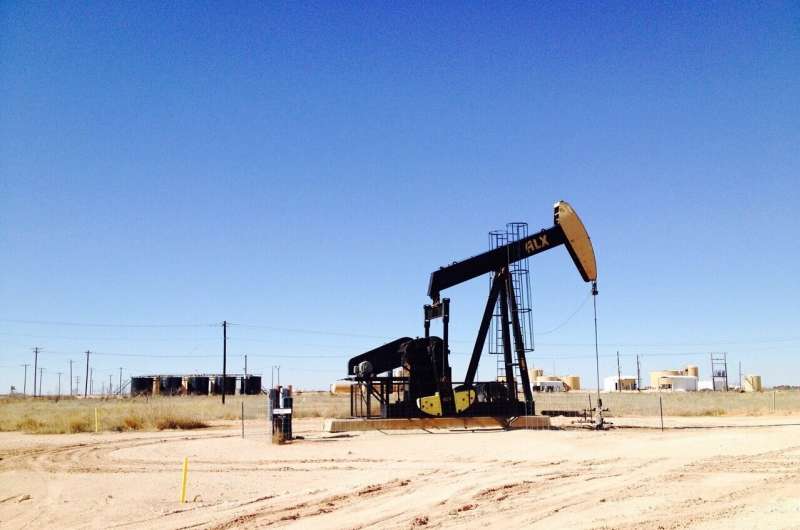
Researchers from Skoltech and Gazpromneft-STC have patented a technique that uses machine learning to get the most out of hydraulic fracturing, a technology employed by oil and gas companies to maximize well production. Based on the intelligent analysis of meticulously collected data on thousands of wells, artificial intelligence makes custom predictions as to what the optimal fracturing parameters for any given well are. Registered by the Russian Federal Service for Intellectual Property, the invention had previously been presented in a January study and one earlier paper in the Journal of Petroleum Science and Engineering.
Hydraulic fracturing involves injecting a high-pressure mixture of water, sand or gravel, and chemicals into the oil-bearing rock formation surrounding a well to increase recovery. It has come to be a fairly routine operation for any new well, but it is not a one-size-fits-all technique. Rather, engineers have to fine-tune a number of governing parameters that have to do with the composition and volume of the injected fluid, pumping rate, loading of particles, and other technicalities.
The conventional approach to setting these parameters relies on complex, time-consuming modeling. Skoltech Professor Andrei Osiptsov, the scientific supervisor of the project, explained: “Educated guesses about what parameters might work best are fed into a physical model of the fracturing process, with the model making predictions about how a given combination of parameters would affect well productivity. This is a very computationally intensive task, and it takes days to weeks to determine the right parameters for just one well, of which there are many at any given oil field.”
“Instead, we train artificial intelligence to predict well production using our very own database—one of its kind!—that lists as many as 92 features of the well, the surrounding rock, and fracking design for about 6,000 wells in 23 oil and gas fields,” Skoltech Ph.D. student Anton Morozov commented. Since the yield of these wells after hydraulic fracturing is already known in retrospect—and is also recorded in the database in the form of 16 more parameters—AI can tie the two together and learn to predict well production that will result from certain initial conditions.
“Once we can predict well production from fracturing parameters, we can actually solve what’s known as the inverse problem: Given certain fixed well and rock characteristics, what fracturing parameters will lead to the highest cumulative production?” project manager, Senior Engineer Albert Vainshtein of Skoltech, explained, saying that AI and optimization algorithms provide the answer to this question very quickly. “The technique is applicable to any oil well, and it has been successfully tested in the Priobskoye field in Western Siberia,” Skoltech Ph.D. student Dmitry Popkov added.
“You can also further enhance this solution to promote more environmentally aware and responsible fossil fuel recovery—putting the ‘E’ in ESG,” Skoltech Ph.D. student Viktor Duplyakov commented. That is, rather than maximizing hydrocarbon production no matter what, the system can be trained to balance yield with environmentally relevant metrics, such as the amount of freshwater consumed, chemicals injected, and diesel burnt on pumps and greenhouse gases emitted. “Perhaps one could even conceive an integrated coefficient indicating the combined ecological or energy effective footprint of a hydraulic fracturing job,” Egor Shel from Gazpromneft Science and Technology Center suggested, with a view to possible future research.
Scientists use machine learning to optimize hydraulic fracturing design for oil wells
V.M. Duplyakov et al, Data-driven model for hydraulic fracturing design optimization. Part II: Inverse problem, Journal of Petroleum Science and Engineering (2021). DOI: 10.1016/j.petrol.2021.109303
Anton D. Morozov et al, Data-driven model for hydraulic fracturing design optimization: focus on building digital database and production forecast, Journal of Petroleum Science and Engineering (2020). DOI: 10.1016/j.petrol.2020.107504
Citation:
AI on big field data helps make hydraulic fracturing more economically viable (2022, July 27)
retrieved 27 July 2022
from https://techxplore.com/news/2022-07-ai-big-field-hydraulic-fracturing.html
This document is subject to copyright. Apart from any fair dealing for the purpose of private study or research, no
part may be reproduced without the written permission. The content is provided for information purposes only.
For all the latest Technology News Click Here
For the latest news and updates, follow us on Google News.